Apr 2025 Edition
Continue readingFrontWell Solutions and TCG Digital Forge Strategic Partnership to Accelerate AI and Analytics Innovation
- April 17, 2025
Bad Vilbel, Germany
FrontWell Solutions, a dynamic and rapidly growing IT solutions provider with focus on Life Sciences and TCG Digital, a global leader in data intelligence & AI enabled business transformation, are pleased to announce a strategic collaboration to drive innovation in the fields of AI and advanced analytics to drive autonomous, data-driven operations.
Why This Partnership Matters
The alliance combines FrontWell’s expertise in integrating and digitizing complex Life Sciences’ production and lab environments with TCG Digital’s mcube™ platform.
mcube™ unifies and activates data from MES, LIMS, MOM and other systems, transforming operations into self-learning, insight-rich environments. This integration accelerates digital maturity, enabling organizations to confidently advance towards AI-powered operations.
What the Partnership Enables
Accelerated Digital Maturity
Agentic AI Capabilities
Ontologies driven intelligence layer
High impact AI applications
This partnership brings targeted value to labs, R&D, and QC environments by enabling high-impact use cases such as predictive formulations, bio-assay AI suite, and AI-led master data management (MDM) for LIMS.
Compliance and Traceability
Accelerated Digital Maturity
Agentic AI Capabilities
Ontologies driven intelligence layer
High impact AI applications
This partnership brings targeted value to labs, R&D, and QC environments by enabling high-impact use cases such as formulation optimization, bio-assay AI suite, and AI-led master data management (MDM) for LIMS.
Compliance and Traceability
A Partnership Built on Innovation and Strengths
This collaboration will also include joint R&D initiatives and co-hosted industry events to advance thought leadership in AI and analytics.

We are not just at the beginning of a new era – we are already amidst transformation. Artificial intelligence is not only revolutionizing our industry but fundamentally changing how our customers think, decide, and operate. This paradigm shift presents tremendous opportunities. Integrating AI into leveraging the Data from the industrial processes is no longer just an option but a strategic necessity. That’s why we are strategically investing in AI and analytics – and we are particularly excited about partnering with one of the leading innovators in this field, TCG Digital.” said Ardavan Heidari, CEO of FrontWell Solutions.

The capabilities of mcube™ in harmonizing complex data and enabling advanced AI-driven decision-making to represent a significant advancement in operational intelligence. This partnership will fundamentally enhance how data is processed and utilized across labs and production lines, increasing reliability of production processes manifold, driving improved efficiency and compliance.” said Arunava Mitra, VP, TCG Digital.
FrontWell Solutions is an expert in the digital transformation of the Life Sciences manufacturing process. Our team of experts is engaged in providing digital solutions to 12 of the 20-leading pharmaceutical, biotechnology, chemical, and medical device companies and suppliers spanning Europe, the United States, and Asia.
Our expertise lies in delivering specialized consulting services, primarily centered around Manufacturing Execution Systems (MES), Laboratory Information Management Systems (LIMS), seamlessly integrating these Level 3 systems with Enterprise Resource Planning (ERP) platforms and driving Manufacturing Intelligence initiatives such as Overall Equipment Effectiveness (OEE) reporting.
TCG Digital is the digital & AI arm of The Chatterjee Group (TCG), a multi-billion dollar conglomerate with a diverse portfolio in Pharmaceuticals, Biotech, Petrochemicals, Aviation, Process Manufacturing across the US, EU, and South Asia. Our umbrella includes companies such as LabVantage, Lummus Technology, and TCG LifeSciences. At TCG Digital, we are driven by our mantra of delivering “Velocity to Value”, helping enterprises achieve topline growth and margin enhancements while strengthening their bottom-line. Our AI Analytics platform mcube is at the heart of these transformations. We enable organizations unlock the full potential of their data, and by seamlessly integrating AI/ML capabilities into their business processes, we empower them to accelerate their digital transformation journey, enhancing agility and driving impactful results.
Media Inquiries:
Julia Tantzky
Marketing & Communications Coordinator
Email: julia.tantzky@frontwell-solutions.com
Phone: +49 170 1789 519
Aditi Basu
Senior Director – Global Marketing
Email: Aditi.Basu@tcgdigital.com
Phone: +919830054094
mcube Details
Hybrid GenAI Search – Combining RAG & Knowledge Graph
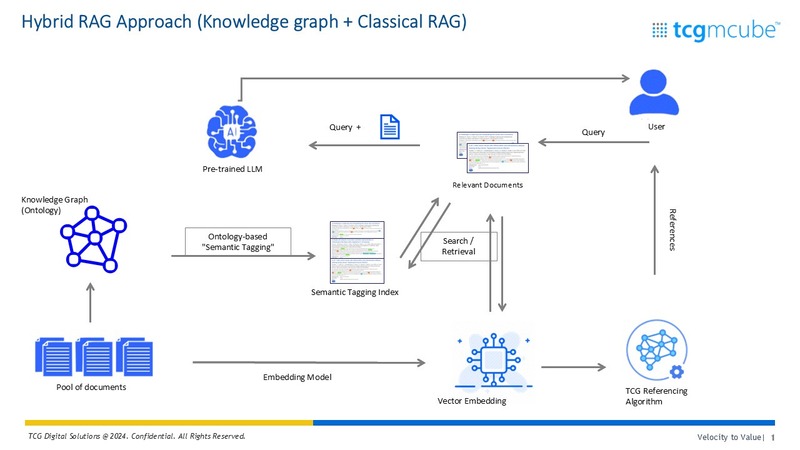
Test Case Creation using GenAI – LIMS Test Method Validation
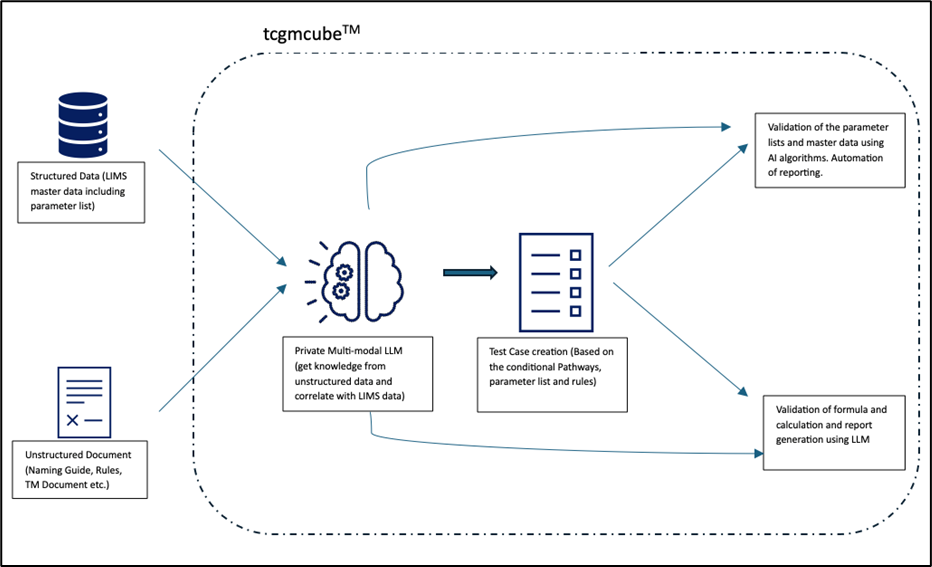
The LLM plays a crucial role by interpreting diverse data sources—such as text documents, method protocols, technical standards, and structured data within LIMS—allowing it to cross-validate test methods, parameter lists, and configurations. This intelligent automation ensures that the entire process adheres to industry standards, improving operational efficiency and accuracy.
Test Method and Naming Verification: The solution begins by validating naming conventions and parameter lists for test methods by analyzing the Naming Guide and comparing it with LIMS metadata. The LLM flags inconsistencies, ensures all required fields (e.g., Parameter List ID, Repeat Count, Equipment links) are completed correctly, and confirms compliance with naming standards, reducing human errors and maintaining procedural accuracy.
Parameter and Configuration Validation: The solution extracts relevant parameters (e.g., Data Type, Units, Replicates) from the test method documentation and compares them to LIMS configurations. This guarantees that the parameter structures align with the test method flow, ensuring data consistency. The LLM also automates sample creation in LIMS to validate data integrity and alignment.
Consumables and Instrument Integration: The multimodal LLM interprets the test method documents to identify the required consumables and instruments, verifying their availability and status (active or expired) within LIMS. This ensures that only available, compliant items are used in test setups, further enhancing accuracy and efficiency.
Validation of Conditional Statements and Calculations:
One of the most complex tasks involves verifying conditional logic and calculated components within test methods. The LLM extracts parameters, rules, and conditions from LIMS master data and generates test data to validate various pathways. Through simulations (using Design of Experiment or LLM methods), it checks for boundary conditions and exception cases, ensuring that calculations and workflows operate as expected.
Unit Consistency and Standards Compliance:
The LLM ensures that the units in Lab Vantage result parameters match the technical standards, preventing errors caused by incorrect units. In cases requiring manual calculations, such as using the Master Verification template, the LLM automates complex calculations, generates reports, and uploads them as evidence for documentation.
In summary, this end-to-end automated solution provides seamless test method validation in LIMS by integrating AI to handle both structured and unstructured data. Its ability to validate workflows, interpret complex calculations, and ensure regulatory compliance significantly reduces human error, boosting efficiency and ensuring accurate test method verification.
RAG – Enterprise Data Search
Our Retrieval-Augmented Generation (RAG) product revolutionizes enterprise search by transforming unstructured data into a searchable knowledge base. With advanced parsing, embedding creation, hosting LLMs privately and a dynamic user interface, this tool empowers businesses to extract insights from vast unstructured datasets with ease.
To battle the hallucination and boost the credibility of generated answers this TCG RAG solution also provides the references to the answers being generated. Hosting LLMs privately ensures that your data and IP is secure.
GenAI-Powered Fabric Design
Leverages Generative Adversarial Networks (GANs) and Neural Style Transfer to create unique fabric that blend traditional aesthetics with modern AI technology.
Neural Style Transfer Algorithm:
- Merges content images (base fabric design) with style images (textures, color schemes, patterns).
- The content image provides structure and layout, while the style image contributes aesthetic features.
- The algorithm minimizes the difference between the original images and the generated design, preserving the fabric’s base structure and integrating artistic styles.
- Allows image uploads for both content and style images.
- Provides sliders to adjust the ratio between “content contribution” (retaining original design) and “style contribution” (influencing the design with style elements).
- Designers can preview real-time iterations, experimenting with different settings before finalizing a design.
- Utilizes StyleGAN for high-quality image synthesis.
- Uses Cycle GAN for unpaired style transfer, enabling transformations without the need for paired data.
- Fabric image collation, pre-processing, image classification, model training, validation, and a design feedback loop.
- Enables designers to create high-quality synthetic saree samples that blend tradition with cutting-edge innovation.
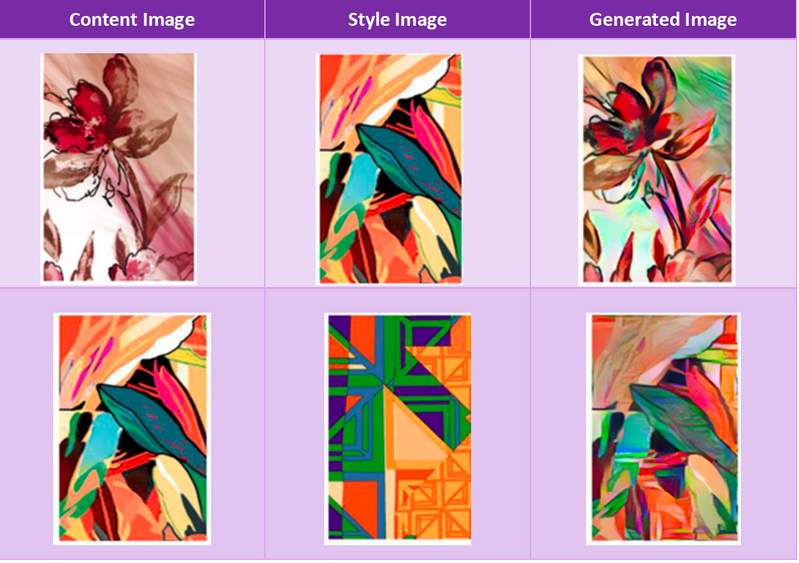
Accelerating Catalyst Formulation with Generative AI
Hydrocracker catalysts are carefully optimized for selectivity vs. activity, balancing these properties to achieve high efficiency in refining processes. These catalysts typically consist of three key ingredients: amorphous Si/Al, crystalline Si/Al, and base metals. To accelerate the formulation process, a combination of Generative AI, Design of Experiment and optimization models is employed:
LLM Fine-tuning for Knowledge Retrieval:
The first step involves fine-tuning a Large Language Model (LLM) on domain-specific documents and research papers related to catalyst formulation. This accelerates the knowledge retrieval process, allowing experts to quickly explore various ingredient combinations. By integrating historical data, the LLM can suggest optimal ratios of amorphous Si/Al, crystalline Si/Al, and base metals, while rejecting infeasible combinations early in the process. This dramatically reduces the time spent on trial-and-error formulations.
Hypothesis Generation with DOE and AI Validation:
The next step is hypothesis generation through Design of Experiments (DOE), where multiple combinations of the catalyst ingredients are generated. The LLM, fine-tuned for the catalyst domain, is then used to assess these combinations, eliminating infeasible hypotheses before they move forward to experimental validation. This AI-driven pre-screening ensures that only the most promising formulations are tested, saving time and resources.
Optimization Model for Selectivity and Activity:
To fine-tune the catalyst for optimal selectivity and activity, historical experimental data is utilized to build an optimization model. This model considers previous test results and the properties of the ingredients, helping to refine the formulation process. The goal is to achieve the best balance between selectivity and activity, ensuring that the catalyst performs efficiently under the specific conditions of hydrocracking.
Agentic Architecture – AI Agents and Text-to-Query Agents
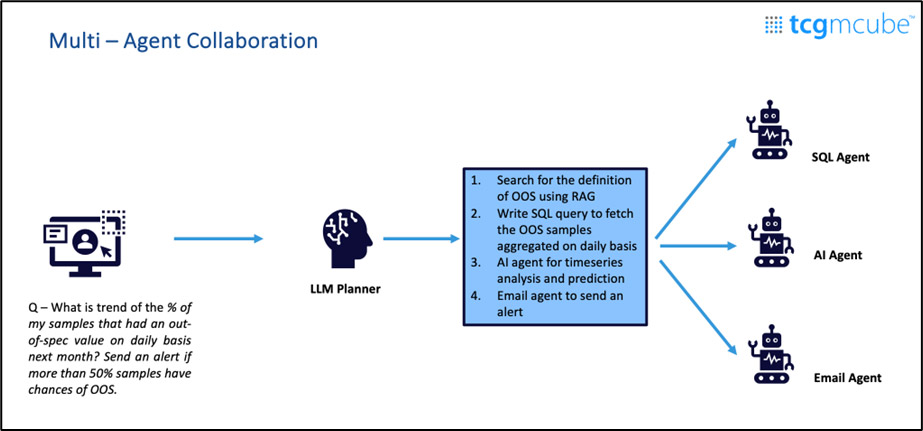
Agentic AI for plant reliability:
Autonomous agents enhancing plant reliability by monitoring asset health, predicting failures, and optimizing maintenance workflows for uninterrupted operations.
LLM for Alert Criticality Analysis:
The first step involves leveraging a Large Language Model (LLM) to analyze the criticality of alerts and correlate them with operational and trip points. The LLM helps assess the urgency and impact of potential anomalies, enabling operators to prioritize and respond to alerts more effectively. This integration enhances the ability to detect anomalies before they escalate, improving overall system reliability.
Identification of Potential Anomalies:
The system continuously monitors data, identifying potential anomalies that could cause slowdowns or shutdowns. By leveraging AI, it flags abnormalities early, helping operators prevent costly disruptions. This early identification allows for timely intervention, minimizing unplanned downtime and ensuring smoother operations.
Alert Importance, Causes, and Outcomes Assessment:
The architecture assists operators in assessing the importance, causes, and potential outcomes of each alert. By correlating the alerts with operational trends and past incidents, it provides a clearer understanding of which anomalies pose the greatest risk. This allows for more informed decision-making and resource allocation when addressing critical issues.
Financial Overlay for Cost and Loss Insights:
The system not only identifies anomalies but also provides insights into the associated costs and losses. By integrating a financial overlay, it quantifies the opportunity cost/production loss and the cost of repairs required to address the anomaly. This dual focus on operational and financial impact enables a more comprehensive approach to risk management.
GenAI agent for Automated Fault Tree Traversal:
To further enhance anomaly resolution, Generative AI is used for automated and guided fault tree traversal. This technique helps identify the root causes of potential anomalies and recommends appropriate corrective actions. By simulating various scenarios and tracing the potential consequences, the system provides actionable insights to mitigate risks and eradicate the threat of future anomalies.
TCG Digital Elevates Football Coverage with Intelligence-Driven Innovations in DFB-Pokal Semi-Final
TCG Digital Elevates Football Coverage with Intelligence-Driven Innovations in
DFB-Pokal Semi-Final
- April 4, 2025
TCG Digital transforms football coverage with real-time multilingual reports, instant match highlights, and inclusive subtitles for Indian fans.
The DFB-Pokal 2024-25 Semi-Final between VfB Stuttgart and RB Leipzig was a thrilling encounter that redefined how football is experienced. Fans across India witnessed a new standard in sports coverage, powered by TCG Digital’s intelligence-driven solutions.
Leveraging mcube™, TCG Digital’s data intelligence platform, this initiative introduced four key innovations: instant multilingual match reports, real-time translations, automated highlight reels, and accessibility-driven subtitles – enhancing inclusivity and engagement for fans.
Bridging the Language Gap: Match Reports in 11 Indian Languages
For the first time, football fans in India could access match reports in 11 Indian languages, including Hindi, Bengali, Malayalam, Marathi, Telugu, Gujarati, Tamil, Punjabi, Assamese, Kannada, and English.
From Angelo Stiller’s opening goal to VfB Stuttgart’s commanding 3-1 victory, every key moment was instantly translated, allowing fans to enjoy the match in their native language. This step brings global football closer to local communities like never before.
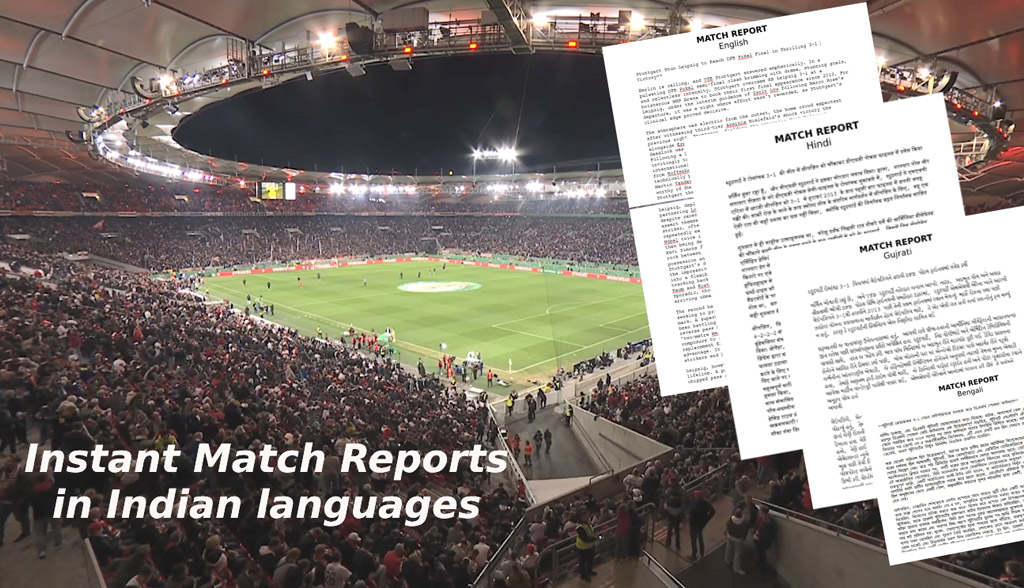
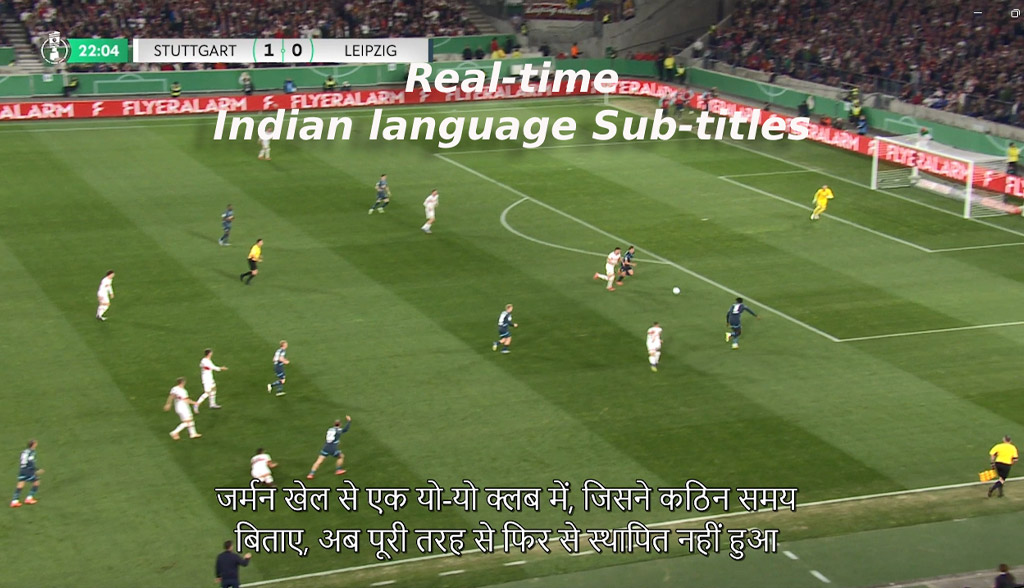
Real-Time Subtitles: Making Football More Inclusive
To make football more accessible, real-time subtitles in Indian languages were introduced, allowing hearing-impaired fans and those who prefer reading along to stay connected with every play.
For instance, as VfB Stuttgart sealed their victory, subtitles in Hindi provided an immersive experience to thousands of fans. With this, sports broadcasting takes a step toward inclusivity, ensuring no fan is left behind.
Instant Match Highlights: Recaps Within One Hour
Understanding the demand for quick post-match analysis, TCG Digital’s intelligence-powered system delivered detailed match highlights within one hour of the final whistle.
This feature ensures football enthusiasts can relive crucial moments instantly, making it easier to stay engaged without waiting for full replays.
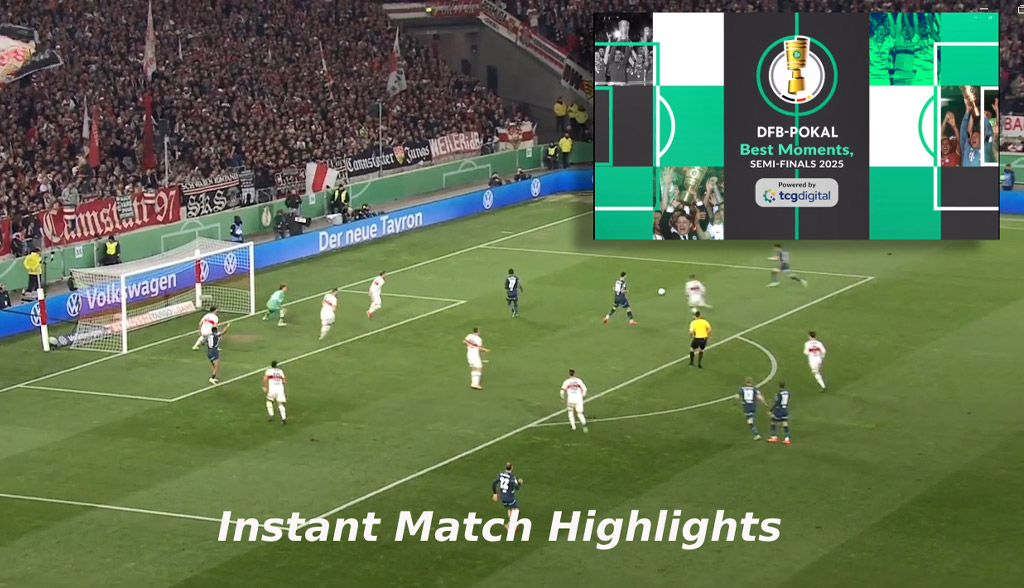
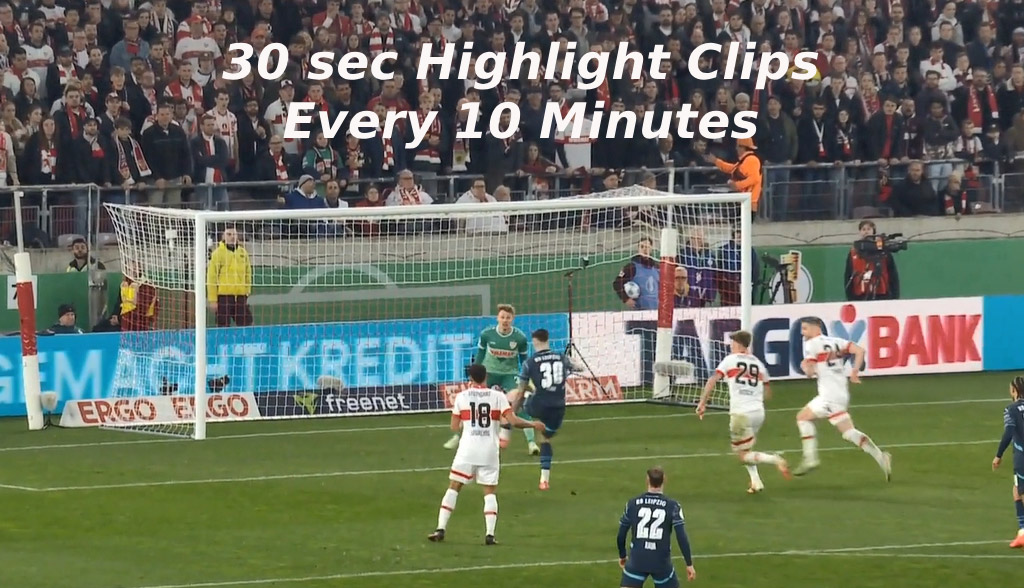
30-Second Highlight Clips Every 10 Minutes
In today’s digital-first sports culture, quick access to key match moments is essential. TCG Digital’s automated system generated 30-second highlight clips every 10 minutes, tailored for platforms like YouTube, Facebook, Instagram, and Twitter.
Fans could instantly share, discuss, and relive match-defining moments, strengthening the connection between the game and its audience.
Statements from Key Stakeholders
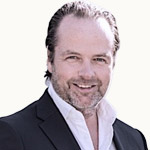
Kay Dammholz,
Director Media Rights, DFB
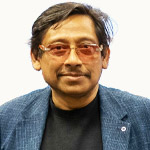
Kaushik Moulik,
Football Philosopher & Social Entrepreneur
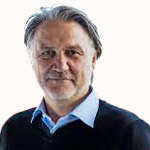
Didi Beiersdorfer,
Former German National Team Footballer
A New Era of Football Engagement
With multilingual accessibility, real-time content automation, and seamless fan engagement, this initiative marks a major shift in football broadcasting.
Football fans in India no longer have to wait for highlights or struggle with language barriers – this innovation ensures instant, immersive, and personalized experiences like never before.
The Deutscher Fussball Bund (DFB) is the governing body of football in Germany. It represents the interests of its member associations at home and abroad and oversees German football’s unity and national teams. DFB GmbH & Co. KG is a wholly owned commercial subsidiary, responsible for marketing, competitions, and strategic growth of German football.
TCG Digital is the digital and intelligence solutions arm of The Chatterjee Group (TCG), a multi-billion-dollar conglomerate with investments in Pharmaceuticals, Biotech, Petrochemicals, and Real Estate across the US, EU, and South Asia.
With its “Velocity to Value” approach, TCG Digital accelerates digital transformation, enabling businesses to maximize the potential of their data. Its intelligence platform, mcube™, is at the core of this transformation, delivering smarter insights and enhanced agility.
Media Contact
Kay Dammholz
Director Media Rights
Email: kay.dammholz@dfb.de
Phone: +49 151 16788 550
Aditi Basu
Global Marketing Director, TCG Digital
Email: aditi.basu@tcgdigital.com
Phone: +49-15257303913 | +919830054094
The Pulse of tcgmcube™ March 2025
Mar 2025 Edition
Continue readingThe Pulse of tcgmcube™ February 2025
Feb 2025 Edition
Continue readingFrom R&D to Manufacturing: How Gen-AI Bridges the Gap for Seamless Tech Transfers in Biopharma
From R&D to Manufacturing:
- February 4, 2025
Introduction
Generative AI (Gen-AI) has emerged as a powerful ally in addressing these complexities, harmonizing cross-functional collaboration, and reducing the risk of data misinterpretation. By creating a single source of truth, Gen-AI not only expedites scale-up but also helps maintain strict regulatory and quality standards.
The Growing Need for Seamless Tech Transfer
Despite these collaborations, misalignment in data standards and processes remains a problem. Lack of common terminology and inconsistent data sharing frequently lead to communication breakdowns and errors. Surveys show that a growing number of biopharma companies outsource at least some of their activities, yet outsourcing alone cannot overcome poor handoffs. That’s where Gen-AI steps in, automating knowledge capture and streamlining the flow of information.
How Gen-AI Bridges the Gap

Knowledge Management and Transfer

Predictive Modeling and
Scale-Up

Real-Time Monitoring and Quality Control
Real-World Impact
How tcgmcube Helps Navigate Tech Transfer Complexities

End-to-End Data Integration
By unifying information from lab notebooks, manufacturing execution systems, and quality management systems, tcgmcube creates a single source of truth. This prevents knowledge silos and ensures all teams—R&D, process development, and manufacturing—stay aligned.

Semantic Models and Knowledge Graphs

Predictive Analytics and
“What-If” Simulations

Regulatory Compliance and Traceability
By eliminating repetitive paperwork, consolidating intelligence, and providing granular control over process parameters, tcgmcube dismantles traditional barriers between R&D and manufacturing.
Citations
- IDBS: Challenges in Tech Transfer for Pharmaceuticals
- Biopharm International: Tech Transfer in the New World of New Modalities
- BIO.org: Success Factors in Technology Transfer for Startups
- Deloitte: Supply Chain Tech Transfer Report
- JPMorgan Licensing and Venture Report
- PharmTech: Strategies for Overcoming Tech Transfer Challenges
- Biopharm International: Addressing the Key Pitfalls Hindering Tech Transfer
Conclusion
The Pulse of tcgmcube™ January 2025
Jan 2025 Edition
Continue readingDeutscher Fussball Bund & the Tech Revolution
Deutscher Fussball Bund
& the Tech Revolution:
Transforming the Game!
Frankfurt, Germany / Somerset, USA
January 27, 2025
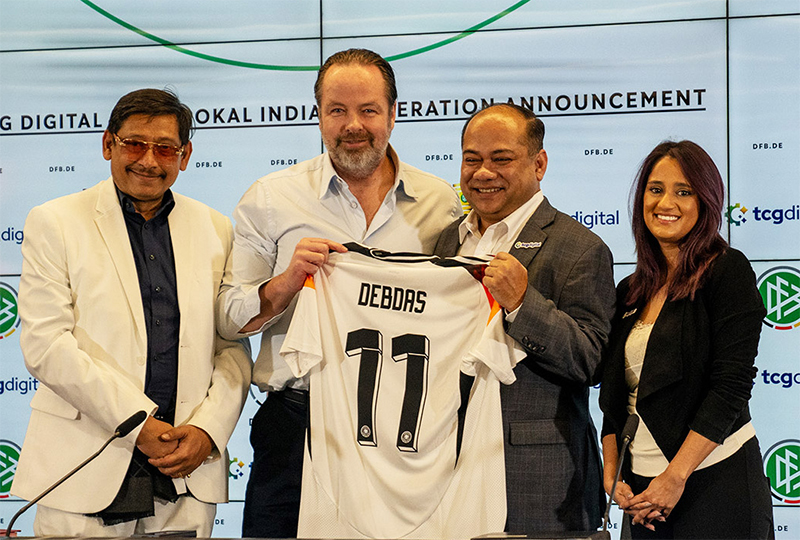
- January 27, 2025
Football is getting smarter, more inclusive, and exciting!
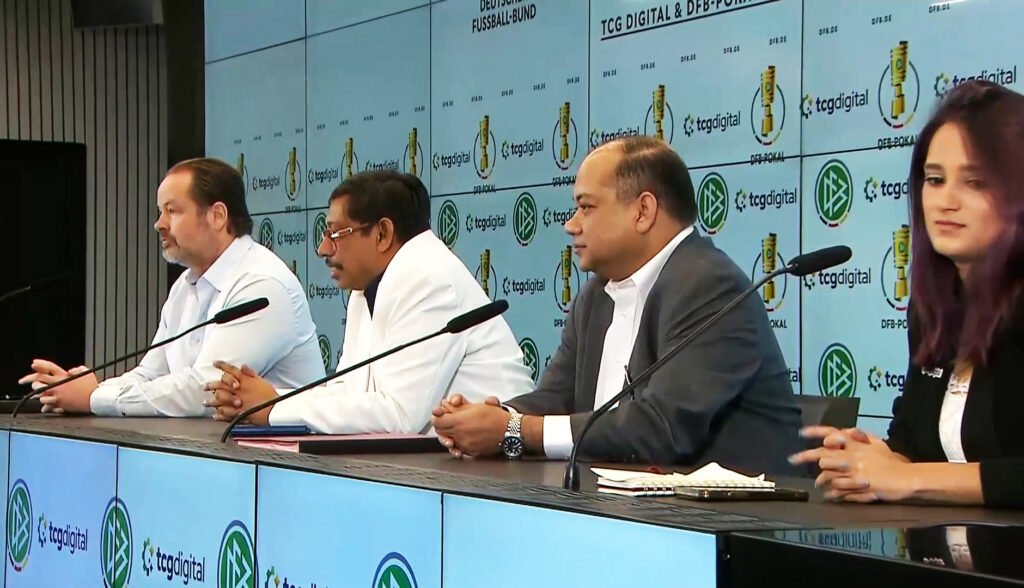
At the heart of this alliance is a pioneering “Co-Innovation Lab” that brings data intelligence & AI into the heart of football. From enhancing fan engagement to potentially scouting the next big talent, this lab is set to seamlessly integrate transformative technology into every facet of the sport. Starting with India and later potentially rolled out to further countries, fans can look forward to live multilingual commentary, automated match intelligence connecting the global community and much more; the unparalleled football archive of DFB may even be made smarter and better for preserving and rejuvenating the game’s rich history.
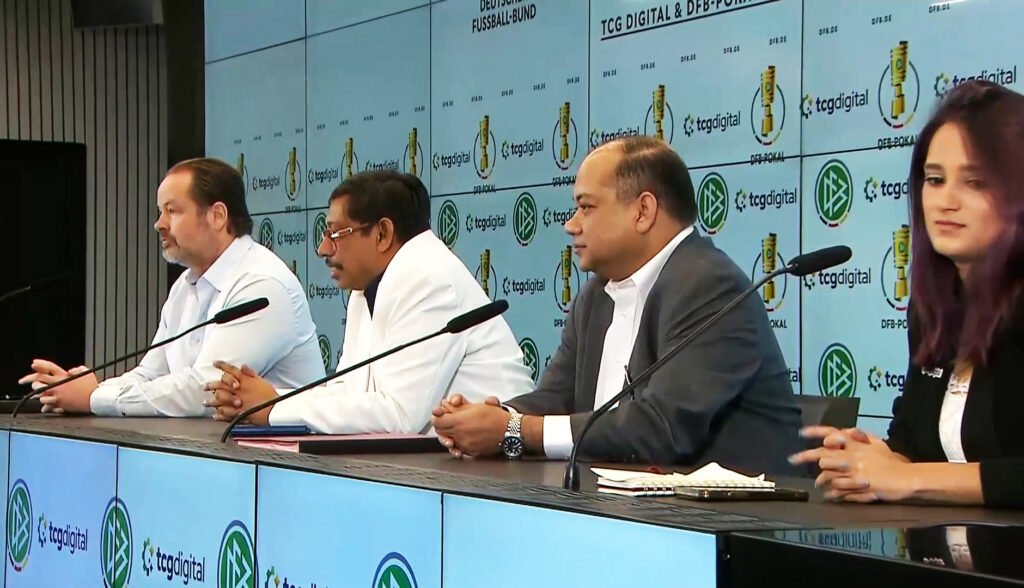
Statements from Our Champions:
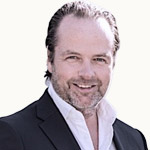
Kay Dammholz,
Director Media Rights, DFB
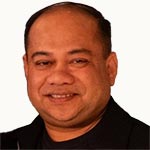
Debdas Sen,
CEO, TCG Digital
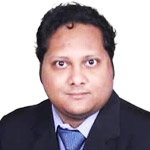
Arunava Mitra,
Vice President EMEA, TCG Digital
For Media Inquiries:
Kay Dammholz
Director Media Rights
Email: kay.dammholz@dfb.de
Phone: +49 151 16788 550
Aditi Basu
Director – Marketing and Press Relations, TCG Digital
Email: aditi.basu@tcgdigital.com
Phone: +49-15257303913 | +919830054094
The Data Lakehouse – Foundation for scaling AI-based innovation across the enterprise
In the era of big data, advanced analytics, and AI, the need for efficient data management systems becomes critical. Traditional data warehousing and data lake architectures have their limitations, particularly in navigating through diverse and voluminous datasets, making it extremely difficult for users to get to relevant, contextualized data.
Continue reading