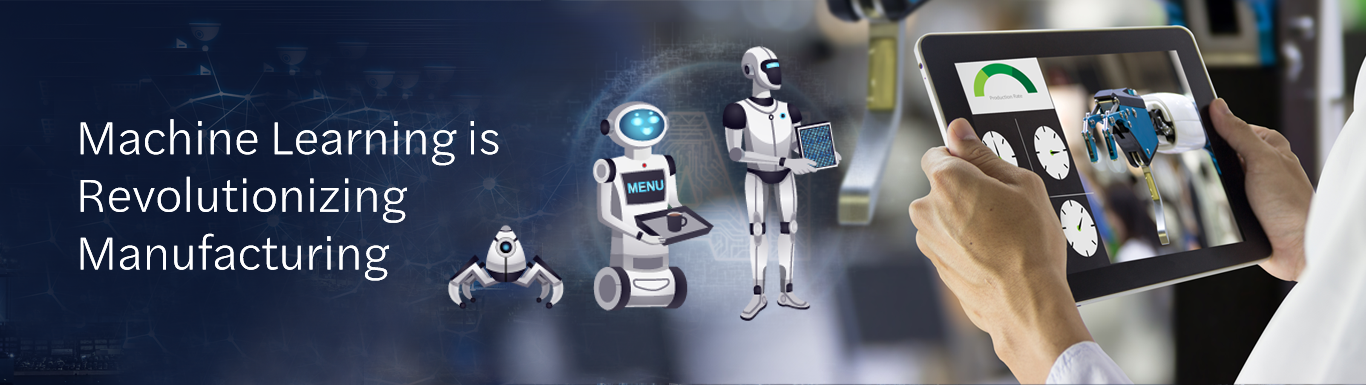
In the prevailing times, the manufacturing process for companies can be cost-intensive and time-consuming if they are not equipped with suitable technologies. One critical and emerging technology which is all set to revolutionize manufacturing is machine learning (ML). From improving productivity to bringing about efficiency gains, ML algorithms have been seen to transform each step of manufacturing. These algorithms reduce the length and cost of manufacturing processes and harness the potential of varied datasets to make smart manufacturing a reality. They collect data from the manufacturing environment and use sophisticated and advanced computing power to arrive at actionable insights.
According to the predictions of a leading market intelligence provider, the smart manufacturing market will be worth $320 billion by 2020 and grow at a projected CAGR of 12.5%. Now, what is smart manufacturing? As most of us are aware that Industry 4.0 is underway, there is a shift from traditional manufacturing to smart manufacturing where machines are trained to understand processes and robots assemble a product with high levels of precision. This primer provides us with insights into how ML finds significant use in modern-day manufacturing.
ML methods used in manufacturing
The three types of ML methods used in manufacturing include supervised, unsupervised and reinforcement learning. Let’s understand each of the three with use cases:
Supervised learning
Supervised learning is an ML technique where large training data sets are applied to the systems. The system is trained with data that has already been categorized into one or more groups, and the groups here are referred to as labels. The system makes use of the algorithms to understand the data structure and then classifies the output data under the right category. Let’s take the Statistical Learning Theory (SLT) as a case in point. Bayesian Networks are the most well-known application of SLTs. An American multinational information technology company in Corvallis, Oregon manufactures numerous precision products at immensely high speed. One essential component in the production of these products was the alignment of the cap to the base. Hence, the positional accuracy of the cap was critical. The company developed a prototype to monitor the performance of the alignment process in real-time. For the monitoring model, they used Bayesian networks which led to positive results.
Unsupervised learning
In the case of unsupervised learning, the evaluation is not dependent on pre-classified labeled data. Instead, the algorithms detect patterns in the unclassified data and these groups of related observations are called clusters. The final objective of unsupervised learning is to decipher unknown but evident relationships between the clusters. Clustering is a common example of unsupervised learning which is being adopted by companies such as Acta-Mobilier which provides high quality lacquered furniture. The company was grappling with rework rates almost as high as 30%. This resulted in a significant gap between the increasing customer requirements and the speed of implementing new processes. The company implemented clustering algorithms to address this production logistic problem which eventually reduced the rework rates.
Reinforcement learning
In addition to supervised and unsupervised learning, manufacturers also adopt reinforcement learning. Take for instance, a world leader in industrial robotics which integrates deep reinforcement learning into its industrial robots. Towards the end of 2016, an American tech major collaborated with this leader in factory automation to use the latter’s AI chips in the Smart Factory for efficiency gains.
While the use of ML algorithms will only be on the rise, there are some key areas where the transformation is already evident:
In supply chain management
It is interesting to note that according to a Gartner study, by 2020, 95% of supply chain vendors will depend on supervised and unsupervised learning. ML algorithms provide supply chain operators with significant insights into how to improve the supply chain performance, anticipate the anomalies in logistics, and identify areas that can be automated leading to scale advantages. ML is revolutionizing supply chain management in four key ways:
- Reducing logistics costs
- Detecting inconsistent supplier quality levels
- Reducing risk and potential for fraud and;
- Providing end-to-end supply chain visibility
In predictive maintenance
Predictive maintenance is another critical area in manufacturing which ML is fast transforming. One of the Big Four accounting firms anticipates that implementation of ML and analytics by manufacturers will rise by 38% in the next five years only to enhance predictive maintenance.
ML specifically helps in:
- Remaining useful life (RUL) prediction: In this case, the algorithms give insights into when a machine will fail so that maintenance can be scheduled well in advance. Regression is used to calculate the RUL of an asset.
- Anomalous behavior: Anomalies are detected through time series analysis.
- Failure diagnosis: ML algorithms are used to diagnose likely failures beforehand. The algorithms also recommend mitigation and maintenance actions after the failure.
The use of ML in predictive maintenance can be understood better with a real-life use case. A manufacturer of industrial equipment in the beverage industry integrated the existing machines with an ML-based monitoring and prediction system. This reduced problems of inefficiency and reactive customer service. It also helped optimize the equipment maintenance schedules which took place at defined time intervals previously and did not cater to maintenance needs in real-time. Eventually, the use of the ML-based prediction system led to business scalability and optimized the cost structure.
In quality control
Lastly, quality control is another function where ML finds significant use. According to a prediction by Forbes quality testing done with machine learning can boost detection rates by 90%. Machine learning allows algorithms to inspect products and identify flaws. These ML-based algorithms make use of samples in the training set and create a library of the possible defects. Further, they learn from samples to distinguish the faultless from the flawed.
As ML heralds a new age of predictive manufacturing, optimized supply chains, and enhanced quality control, it will also mean a quantum leap to make Industry 4.0 a reality. What’s more, machine learning being a self-learning system will only lead to better outcomes resulting in improved production throughput at superior quality levels.